Flexible Modulation of Neural Variance Facilitates Abstract Skill Learning
Introduction
One hallmark of natural motor control is the brain’s ability to adapt to perturbations ranging from temporary visual-motor rotations to paresis caused by stroke. These adaptations require modifications of learned neural patterns that can span the time-course of minutes to months. Previous work with brain-machine interfaces (BMI) has shown that over learning, neurons consolidate firing activity onto low-dimensional neural subspaces, and additional studies have shown that neurons require longer timescales to adapt to task perturbations that require neural activity outside of these subspaces. However, it is unclear how the motor cortex adapts alongside task changes that do not require modifications of the existing neural subspace over learning.
Methods
Five nonhuman primates were used in three BMI experiments, which allowed us to track how specific populations of neurons changed firing patterns as task performance improved. The typical closed-loop BMI paradigm is shown in Figure 1A. Neural activity was recorded from motor cortex and spiking activity was binned in 100 ms chunks before being fed into a decoder. In each experiment, neural activity was transformed into cursor kinematics using decoding algorithms such as Kalman filters that were periodically readapted based on natural arm movements or visual feedback. In each experiment, animals were instructed to move a computer cursor from a center target to one of eight peripheral targets. Rewards were given after animals successfully held the cursor within the target location for 200 ms (Figure 1B).
Experiment 1 (Figure 1C, green) consisted of switching between two decoders each day. In Experiments 2 and 3 (Figure 1C, blue and purple, respectively), decoders were readapted multiple times throughout the learning process. Moreover, Experiment 3 consisted of readapting the decoder each day and consisted of controlling a kinematically redundant virtual arm, but otherwise the task structure was the same as the other two experiments.
Experiment 1 (Figure 1C, green) consisted of switching between two decoders each day. In Experiments 2 and 3 (Figure 1C, blue and purple, respectively), decoders were readapted multiple times throughout the learning process. Moreover, Experiment 3 consisted of readapting the decoder each day and consisted of controlling a kinematically redundant virtual arm, but otherwise the task structure was the same as the other two experiments.
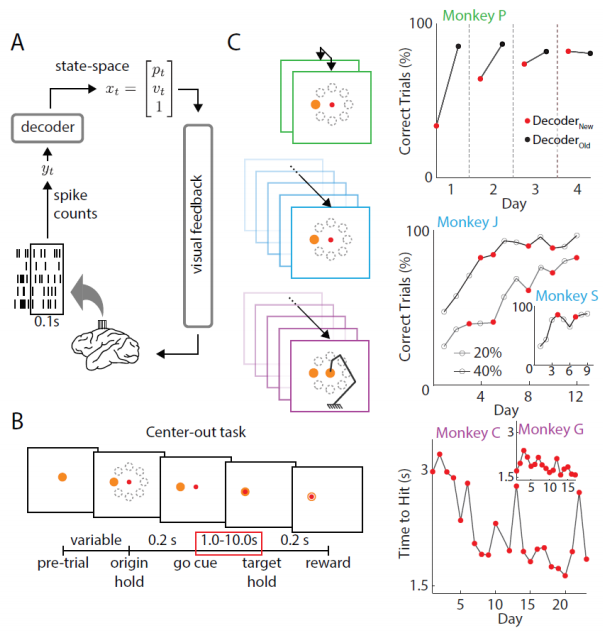
Figure 1. A. Neurons were decoded from motor cortex and transformed into cursor velocities using Kalman filters. B. Trials were self-initiated whereby subjects moved a cursor from a center target to a peripheral target for a juice reward. C. In each of the three experiments, performance improved over time. Red dots indicate when a decoder swap occurred. In Experiment 2, 20% and 40% indicate the initial performance levels with sub-optimal decoders. Decoders were readapted over days to better fit the neural intentions. Experiment 3 shows the reach times since Correct Trials (%) was already saturated on Day 1.
Results
In each of the experiments, we observed improvements in performance over days (Figure 1C). Red dots indicate when decoders were readapted. In Experiment 3, the percent accuracy was already saturated on Day 1. However, reach times continued to improve as animals learned to control the BMI.
We were first interested in how neurons consolidated activity over learning. To do this, we used a factor analysis (FA) model to decompose the variance in the data into coordinated (shared) variance and independent variance (private). Intuitively, shared variance corresponds to the covariance that exists among the entire subpopulation of neurons we're recording from while private variance corresponds to the variance each neuron has that is uncorrelated with the rest of the population. For more detailed methods on the analysis, please refer to Athalye et al., 2017.
Consistent with past results, we found that the proportion of total variance captured in the shared spaces (SOT) increased as performance improved for all five animals, indicating a consolidation effect over learning (Figure 2 B, D, F). Notably however, this consolidation occurred over many days. In contrast, the private variance fluctuated on shorter timescales (Figure 2 A, C, E). Each time the decoder was readapted, private variance increased, suggesting neurons were exploring the neural space. Together, it is clear that there is a decoupling between consolidation and exploration, where both mechanisms can coexist as animals learn to improve their control.
We were first interested in how neurons consolidated activity over learning. To do this, we used a factor analysis (FA) model to decompose the variance in the data into coordinated (shared) variance and independent variance (private). Intuitively, shared variance corresponds to the covariance that exists among the entire subpopulation of neurons we're recording from while private variance corresponds to the variance each neuron has that is uncorrelated with the rest of the population. For more detailed methods on the analysis, please refer to Athalye et al., 2017.
Consistent with past results, we found that the proportion of total variance captured in the shared spaces (SOT) increased as performance improved for all five animals, indicating a consolidation effect over learning (Figure 2 B, D, F). Notably however, this consolidation occurred over many days. In contrast, the private variance fluctuated on shorter timescales (Figure 2 A, C, E). Each time the decoder was readapted, private variance increased, suggesting neurons were exploring the neural space. Together, it is clear that there is a decoupling between consolidation and exploration, where both mechanisms can coexist as animals learn to improve their control.
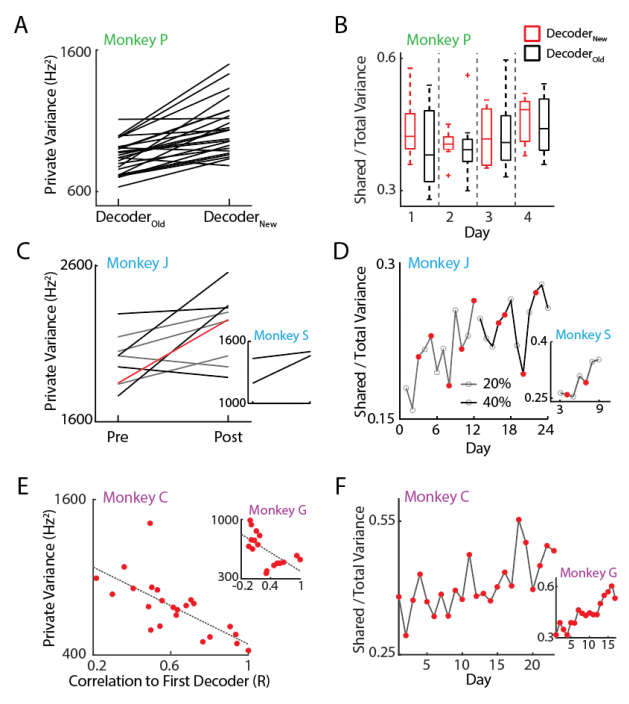
Figure 2. A .Private variance increased each time the new decoder was used. B. The SOT did not appreciably change over the timecourse of 4 days for Experiment 1. C. Private variance increased for Experiment 2 each time a decoder was readapted. The red line indicates the change in private variance when going from the 20% condition to the 40% condition. D. SOT increased over many days. E. Private variance was higher for decoders that were less familiar to the animals. F. SOT increased over days.
Previous work has shown neurons to "rotate" their low-dimensional subspaces over time for large perturbations. Conversely, small perturbations have been shown to not largely affect how these subspaces rotate over learning. To characterize how these subspaces might rotate, we calculated the shared space alignment (Figure 3A) which compares the amount of variance captured in one day's shared space compared to another. In a 2D example, this projection is equivalent to the cosine of the angle between the two planes. Consistent with these results, since our "perturbations" were decoder readaptations to natural movements, we would not expect large changes in the subspace rotations (Figure 3). However, the stability of these planes coexisting with both short and long-timescale changes in neural activity indicates a propensity to modulate neural variance instead of adapting population-level coordination patterns.
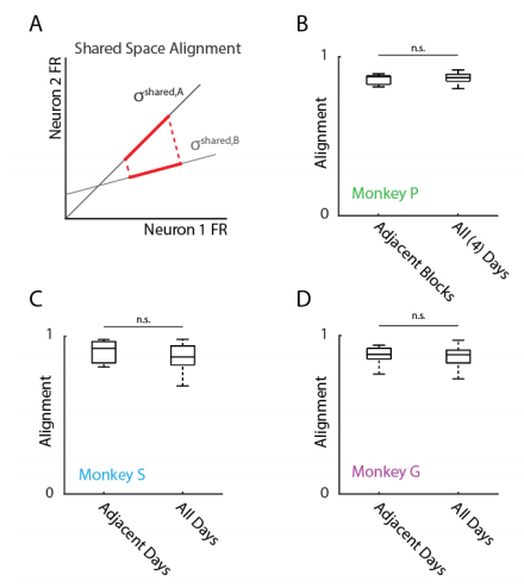
Figure 3. A. Geometric visualization of calculating shared space alignment. The shared space of one block was projected onto the shared space of another. A projection equal to 1 would indicate perfect alignment of subspaces. A projection less than 1 indicates a difference in subspaces. B-D. Alignment values were close to 1 and consistent across days indicating little change in the subspace rotations.
Discussion
Our results indicate that in a BMI system where decoders are periodically refit to the neural intentions of the subject, there are short and long-timescale mechanisms of adaptation. New decoder weights give rise to small changes in decoding for individual neurons, driving private activity to increase. However, these increases in exploratory patterns are not generated randomly. Rather, they are modifications of existing network patterns, which consolidate over multiple days. The concomitant exploration and exploitation of neurons while maintaining a stable subspace highlights the resilience of motor cortex to perturbations.